
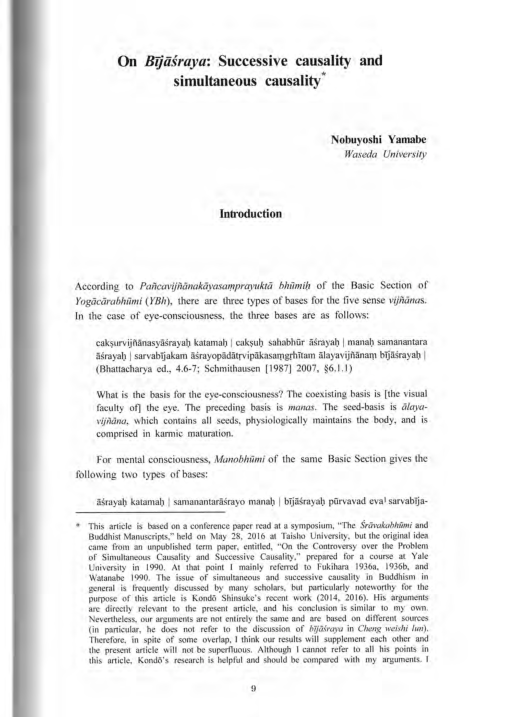
The analyses of data from observational genomics studies are associational by nature, when one looks to make some kind of inference and knowledge discovery from patterns of association using statistical and data mining techniques. The lack of ‘experimental’ interventional data in genomics is mainly due to the logistical feasibility and high cost of their production, which requires genes and proteins experimental perturbations (gene knockout for example). This associational approach based on observational data has dominated genomics studies because of the scarcity of experimental data, where some variables are controlled (fixed to some values) or manipulated and their effects on other variables are measured. The value of large, complex and linkable information generated by genomics and other -omics fields and large scale epidemiologic studies has resulted in the domination of associational reasoning, where scientists look for association/correlation between a large number of diverse and heterogeneous attributes (genes, proteins, polymorphisms, phenotypes).
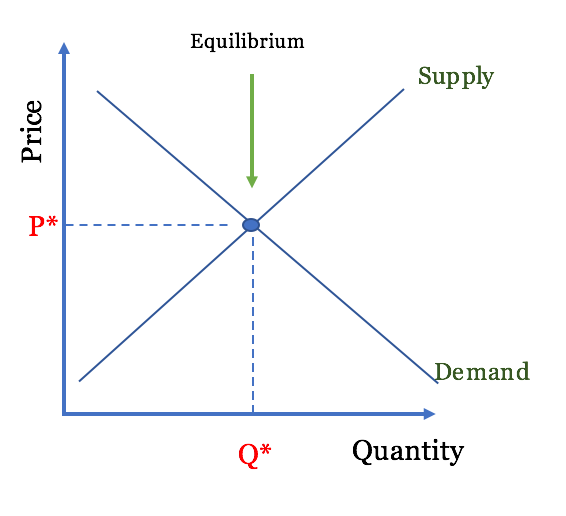
Several paradigms have occurred in successive waves during the last two centuries and they have moved life sciences from empiric to experimental (typically hypothesis-driven) and then to data-driven, concretized by the recent entry in the “big data” era. Biological sciences have been for so long dominated by observational approaches as shown by the scarcity of studies trying to infer causality from association, starting from the historical and very instructive studies of Pierre Louis (1835) on the efficacy of the standard treatments for pneumonia and John Snow (1855) on the causal relationship between cholera and water contamination.
